Benötigte Lesezeit: 6 Minuten
Kathleen und ich werden oft gefragt, wie wir uns den Themen künstliche Intelligenz und maschinelles Lernen angenähert haben. Daher haben wir zusammengefasst, wie uns der Einstieg gelungen ist. Diese Sammlung an Links und Informationen ist nützlich für jeden, der seine Begeisterung für Datenwissenschaft und Programmieren entdeckt hat und dem weißen Kaninchen folgen möchte.
1. Recherche mit Google Scholar
Unsere Begeisterung für künstliche Intelligenz und maschinelles Lernen began im Jahr 2016 als wir die In-Bildsuche von Pinterest entdeckten. Schnell war klar, dass maschinelles Lernen (bzw. maschinelles Sehen) hinter der Technologie der visuellen Suchmaschine steckte. Unsere Begeisterung war so groß, dass wir nach mehr Informationen im Web suchten. Die innere Motivation war ein wichtiger Treiber unserer Wissensreise. Daher kann ich empfehlen, sich einen Bereich oder Anwendungsfall der KI rauszusuchen, der persönlich die größte Neugier erweckt. Dieser ist dann der Startpunkt und das erste Suchwort, um das sich deine Recherchen drehen können.
Eine intrinsische Motivation ist der sicherste Schlüssel zum Erfolg.
Wir begannen, Googles Katalog sämtlicher wissenschaftlichen Abhandlungen zu durchforsten: Google Scholar. Hier entdeckten wir internationale Statistiken, Patente, aktuelle Forschungsarbeiten und Studien zu den Bereichen visuelle Suche, KI oder tiefes maschinelles Lernen (Deep Learning).
- Viele Dokumente sind nur kostenpflichtig einsehbar. Das Googeln des Titels in der regulären Suchmaschine führt oft direkt zum PDF – ganz ohne Registrierung oder anfallenden Kosten.
- Künstliche Intelligenz ist ein global erforschtes und relevantes Thema. Daher ist die englischsprachige Suche wesentlich ergiebiger als die deutschsprachige Suche.
Zu Beginn ließen wir den methodischen Teil der wissenschaftlichen Arbeiten außer Acht. Dieser beinhaltet komplexe mathematische Formeln oder technische Details, die für uns zu diesem Zeitpunkt wenig verständlich waren. Der wertvolle Part für alle Einsteiger ist die Einführung und die Zusammenfassung der Ergebnisse. Hier finden sich die Essenz der Forschung, ähnliche Arbeiten und damit der nächste Schritt im Prozess der Weiterbildung. Wertvoll sind zudem die Quellenangaben, die auf relevante Literatur oder Experten in dem Bereich verweisen. Diese Art der Recherche bietet einen guten Einstieg in praktische Anwendungsfälle, den aktuellen Forschungsstand und führende Köpfe auf dem Gebiet.
Einige Dokumente, mit denen wir uns auseinandergesetzt haben:
- Visual Discovery at Pinterest von Andrew Zhai, Dmitry Kislyuk, Yushi Jing, Michael Feng, Eric Tzeng, Jeff Donahue, Yue Li Du, Trevor Darrell
- Efficient and Privacy-preserving Voice-based Search over mHealth Data von Mohammad Hadian, Thamer Altuwaiyan, Xiaohui Liang, and Wei Li
- Perspectives for Evaluating Conversational AI von Mahipal Jadeja, Neelanshi Varia
Wer automatisch die aktuellsten und wichtigsten Forschungsarbeiten erhalten möchte, dem empfehle ich den Newsletter von topbots.com. Hier bekommen Interessierte regelmäßig einen Überblick über neue Paper und Arbeiten direkt in die Mailbox.
2. Das gute alte Lehrbuch
Wen die Recherche im Web nicht überzeugt, der kann auf das klassische Lehrbuch zurückgreifen. Es gibt eine vielfältige Auswahl für Anfänger, Fortgeschrittene und Experten. Mir persönlich hat das Buch „Coding für Dummies“ gefallen. Es enthält zahlreiche Verweise auf exklusive Online-Trainings, die einen auf sehr unterhaltsame und praktische Weise mit dem Programmieren vertraut machen. Selbstverständlich enthält das Werk auch ein Kapitel über maschinelles Lernen.
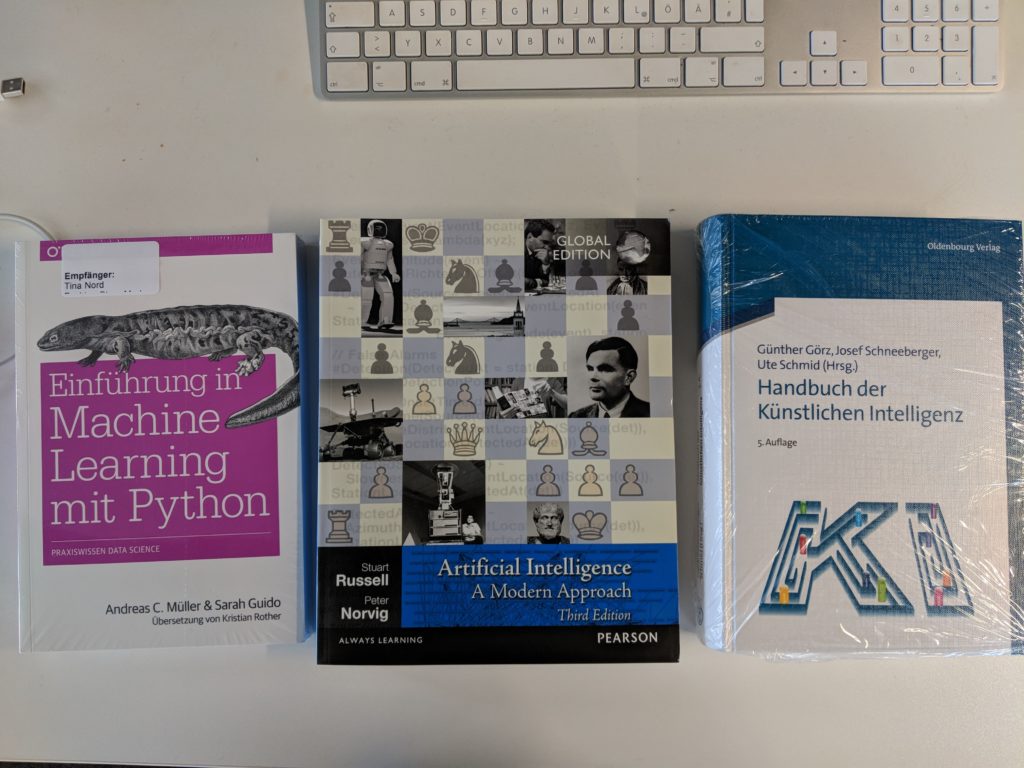
Auf verschiedenen Veranstaltungen holten wir uns zudem Empfehlungen von Professoren ein und erwarben daraufhin folgende Titel:
- „Artificial Intelligence – A Modern Approach“ von Stuart Russell und Peter Norvig
- „Handbuch der Künstlichen Intelligenz“ von Günther Görz, Claus-Rainer Rollinger und Josef Schneeberger
- „Machine Learning mit Python“ von Andreas C. Müller und Sarah Guido
Diese Bücher sind bis heute ein großartige Hilfe bei der Vorbereitung von Vorträgen oder um neue Aspekte des Themas zu erarbeiten. Selbstverständlich gibt es auch Literatur, welche die technischen Details außer Acht lässt und einen praktischen Bezug fokussiert. Dazu gehören zum Beispiel „Weapons of Math Destruction“ von Cathy O’Neil oder das unterhaltsame (und deutschsprachige) Werk von Marc Uwe Kling „Quality Land“.
3. KI Weiterbildungen im Web
Da KI aktuell die Massen begeistert, bieten so ziemlich alle (Elite-) Universitäten Weiterbildungen zu dem Thema an: Harvard, das MIT, Oxford (…). Diese variieren in Bezug auf die Kosten (in der Regel liegen diese zwischen 2.000 und 3.000 Dollar), die benötigte Zeit (6 Wochen) und den Inhalt. In der Regel bieten diese namhaften Institutionen einen guten Überblick über die Theorie des maschinelles Lernens oder künstliche Intelligenz, mit wenigen technischen Details. Eine günstigere Alternative sind private Online-Akademien, wie Udacity, Udemy oder Coursera, die Zugang zu anerkannten Kursen (i.d.R. zwischen 20 und 80 Euro) von z.B. der Stanford University bereitstellen.
Kathleen und ich entschieden uns für das Massachusetts Institute of Technology, da es sich um eine technische Hochschule mit eindeutigem historischen Bezug zur künstlichen Intelligenz handelt. Außerdem fokussierte sich das Training auf Business Use Cases, was uns als Marketing Expertinnen sehr entgegenkam. Wir erhielten einen tief-gehenden Einblick in maschinelles Lernen und lernten Anwendungsfälle kennen. Wesentlich wichtiger war jedoch das Ziel des Kurses, Use Cases selbst identifizieren zu können und eine (Business-)Strategie zur Implementierung von KI zu entwickeln. Notwendig war dazu ein niedriges, vierstelliges Investment, welches sich jedoch lohnte. Zeitlich war der Kurs nebenberuflich umsetzbar.
- Oxford Artificial Intelligence Programme
- MIT Artificial Intelligence: Implications for Business Strategy
- Harvard Online Artificial Intelligence Courses
- Artificial Intelligence Kurse von Coursera
- KI Grundlagen Kurs von Udemy (Deutsch)
Wer stattdessen die praktische Implementierung erlernen möchte, kann dies zum Beispiel für ein kleineres Budget bei Codeacdemy.com tun. Hier gibt es verschiedene Optionen zur Aneignung von Wissen. Ein Anfängerkurs kostet z.B. 20 Euro pro Monat und beginnt mit der Programmiersprache SQL und führt über Python bis hin zum maschinellem Lernen. Wer ein offizielles Zertifikat erwerben möchte, muss 200 Euro & 7 Wochen Zeit investieren und hat dann eine komplette Einführung erhalten. Ich bin persönlich ein großer Fan der Kurse, da die Komplexität langsam zunimmt und die Erfolgserlebnisse pro Lernschritt einen bei Laune halten.
Am Ende ist das benötigte Skillset eine Kombination aus drei Dingen: Allgemeinverständnis für KI und maschinelles Lernen und Basiskenntnisse der Datenwissenschaft sowie einer Programmiersprache wie Python, R+ oder JavaScript.
4. Offline Kurse und Studium
Wir hatten die glückliche Gelegenheit, parallel zur Weiterbildung am MIT einen praktischen Kurs an der Code Universität Berlin absolvieren zu können. Leider ist dieses Angebot nicht regulär buchbar, sondern ein spezielles Programm für Unternehmen und deren Mitarbeiter. In Projektgruppen lernten wir den vollständigen Ablauf der Implementierung von ML kennen: Dies begann mit der Installation von Python, führte über die Definition eines Use Cases und dem Finden einer Datenbasis bis hin zur Recherche und Anwendung von Algorithmen. Anschließend wurde das Resultat in einen Prototypen eingebaut.
Solche umfassenden Kurse sind kosten- und zeitintensiv. Eine Förderung durch den eigenen Arbeitgeber kann daher hilfreich sein. Letzten Endes profitieren Firmen von Mitarbeitern, die den Kontext des eigenen Verantwortungsbereiches mit ML kombinieren und so potenziell die Effizienz steigern können.
Wer ein vollwertiges Studium in Betracht zieht, hat inzwischen mehr und mehr Auswahl. Sowohl lokale als auch internationale Universitäten bieten die verschiedensten Abschlüsse (Bachelor, Master, MBA) und fachlichen Ausrichtungen an. Wer sich früher mit nur einem Semester KI im Informatikstudium zufrieden geben musste, kann sich heute ausschließlich intelligenten Systemen widmen. Obwohl hier gerade in Deutschland noch immer Nachholbedarf besteht, erkennen nahezu alle Fakultäten den Bedarf und beginnen ihr Lehrangebot umzustellen. Wir sind z.B. gespannt auf den Studiengang von Andrew Ng und dem Imperial College London, welcher einen Master in maschinellem Lernen zum Ziel hat und im Herbst 2020 startet.
5. KI Netzwerke und Workshops
Bis dahin gibt es verschiedene Netzwerke, denen du dich auch als Laie anschließen kannst. Dort werden häufig Informationen über Workshops, Hackathons und Konferenzen geteilt und Wissen ausgetauscht. Ein Beispiel ist das internationale und inklusive Netzwerk Women in AI.
Ein regelmäßiger Austausch mit anderen Enthusiasten sichert zudem, dass man nicht den Anschluss verliert. Künstliche Intelligenz entwickelt sich schneller denn je, dank der großen und breiten Begeisterung für das Thema. Weiterbildung ist daher ein nie endender Bestandteil der Arbeit mit KI.
Kennst du weitere Netzwerke oder Weiterbildungsmöglichkeiten? Dann poste deine Empfehlung in den Kommentaren.
Bild: DALLE 2
Great web site! It looks very good! Sustain the excellent work!
Thanks Safia.
I like viewing web sites which comprehend the price of delivering the excellent useful resource free of charge. I truly adored reading your posting. Thank you!
Thank you! We are glad you like our content!
Hallo Tina,
vielen herzlichen Dank für deinen tollen Beitrag!
Bin wirklich sehr gespannt, wie sich noch alles ändern wird mit dem Internet of Things sowie der AI.
Liebe Grüße
Hey Michael, vielen Dank! Wir halten dich gerne weiterhin auf dem Laufenden!